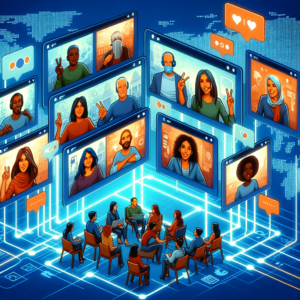
Conversational analytics delves into the analysis and interpretation of natural dialogue, especially in interactions between customers and businesses via platforms like chatbots, virtual assistants, and automated messaging systems. It’s a growing field, projected by Gartner to significantly reduce contact center costs by 2026. As customer engagement increasingly relies on such technology, conversational analytics is becoming vital for effective customer relationship management.
This analytics form aims to comprehend conversation content, context, intentions, emotions, and other critical aspects. Its purpose is to derive actionable insights for better customer experiences, enhanced service quality, and informed managerial decisions.
Natural Language Processing (NLP): This AI branch enables computers to interpret human language, essential for deciphering text or voice inputs in conversational analytics.
Sentiment Analysis: This process identifies the emotional tone in human speech, aiding businesses in assessing customer satisfaction and pinpointing issues.
Intent Recognition: Understanding the customer’s query purpose allows businesses to respond accurately, enhancing conversational effectiveness.
Customer Journey Analysis: Analyzing interactions across various touchpoints provides insights into customer experiences with the business.
Performance Monitoring: Monitoring tools, like chatbot dashboards, help track metrics such as response times and resolution rates, pinpointing areas for improvement.
Topic Extraction: Identifying primary conversation topics helps focus on customer concerns and detect trends.
Personalization and Recommendations: Conversation analysis enables tailored responses and suggestions based on customer behavior and preferences.
Data Collection and Preprocessing: Gathering data from chats, emails, social media, and voice interactions, followed by pre-processing to clean and organize the text.
Processing: Applying NLP to understand conversation content, identifying entities, sentiments, intents, and contexts.
Analysis and Reporting: Using analytics platforms to generate insights and metrics, presented through visualization tools for clear, actionable interpretation.
Conversational analytics faces challenges like the complexity of natural language, context sensitivity, data quality issues, bias in training data, scalability, multilingual support, privacy concerns, and the need for continuous learning and user trust building.
Overcoming these challenges requires ongoing technological advancements and a thoughtful approach to data management and system design. As these hurdles are addressed, the benefits of conversational analytics will become increasingly apparent for organizations. Forward acting companies will readily adopt these exciting new technologies.
Book A Quick 15 Minute Call,
And We’ll Show You How To Unlock The Power Of Every Conversation.
©2024 All rights reserved – Convirza